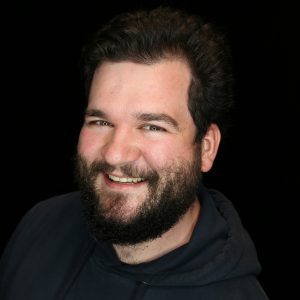
Hannes Engler is a researcher at the institute for grapevine breeding Geilweilerhof from the Julius Kuehn-Institute in Siebeldingen. Here he works in the BMEL founded project: “Artificial intelligence in the vineyard for innovative yield forecasting on grapevines” (KI-iREPro) in the working group for digitalization and precision viticulture. He studied agricultural sciences at the University of Hohenheim.
In viticulture yield is closely linked to the quality of grapes and the product wine and determines the economic viability of a vineyard. In general terms one could say, the higher the yield the lower the wine quality . Yield is therefore one of the most important parameters for wine production and needs to be in a good equilibrium with the production goals. Until now, yield estimation is usually done manually which is time consuming and prone to errors. Yield is influenced by a various parameters like genotype, soil, weather, disease pressure and vineyard management. Estimation errors in viticulture occur to be on average of +/- 30%. The goal of this study is to use artificial intelligence to improve yield forecasts in viticulture and grapevine breeding.
In a first step for a more accurate yield forecast system three main sources of data are taken into account by the artificial intelligence (AI): (1) long-term yield data, (2) environmental data (soil and weather) as well as (3) process management data. In a second step the AI is extended and further improved by sensor-based acquired data. For this purpose, yield-relevant characteristics are recorded directly in the vineyard in real time by the `PHENOboxx`, which is an Embeded-Vision-System. The most important characteristics include the quantification of shoots, bunches and the mass of dormant pruning wood as well as the nutrient and chlorophyll content of the leaves. The `PHENOboxx` is placed on the `Phenoliner`, a converted grape harvester used for data collection and as a phenotyping platform for grapevine research.
During data gathering and validation for step one first inconsistencies in recorded data have been found. The allocation of yield data to individual plots turned out to be very difficult, as this data was not recorded precisely enough for individual plots within the past 16 years. To overcome this issue, yields from one vinegrower from plots with the same variety were aggregated to obtain an adjusted yield per hectare. Existing weather data gathered from the weather station is also very heterogonous over the last 16 years, allocation to single plots is been done by distance to the weather station. Process management data is only available for certain plots.
It can be said that historic data must be screened very carefully before taken into the modeling process. So far, the AI can forecast yields up to 79,3% correctness just by using the historic data without adding any sensor data in addition.
In the long run, sensor data acquisition will improve the AI and needs to be carried out casually during standard vineyard work on the tractor to improve yield forecasts and therefor quality management in viticulture. To get closer to this goal the `PHENOboxx` will be placed on a all terrain vehicle (ATV) in the upcoming season.
Hannes Engler1, Florian Huber², Pascal Gauweiler³, Julius Kraus³, Artem Yushchenko², Volker Steinhage², Robin Gruna³, Katja Herzog1, Reinhard Töpfer1 and Anna Kicherer1
1 Julius Kühn-Institut, Institute for Grapevine Breeding Geilweilerhof, Siebeldingen
2 University of Bonn, Institute for Computer Science 4, Bonn
³ Fraunhofer IOSB, Institute of Optronics, System Technologies and Image Exploitation, Karlsruhe
E-mail of corresponding author: anna.kicherer@julius-kuehn.de