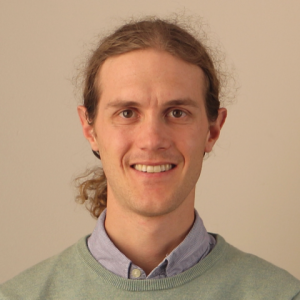
Daniel Runcie is an Assistant Professor in the Department of Plant Sciences at the University of California Davis. Dr. Runcie earned a PhD in Biology and a MS in Statistics at Duke University. His research focuses on methods for identifying and dissecting genetic variation in plants, particularly in traits related to how plants adapt to variation in their environments. Work in his lab spans several plant species from wildflowers to crops, and addresses questions in evolutionary biology, physiology, and plant breeding, and draws primarily on tools from functional genomics, statistics, and quantitative genetics.
Measuring and modeling multiple traits at once can accelerate the rate of genetic gain in breeding programs, whether their goal is to improve a single target trait, or simultaneously improving many traits. Multi-trait data is widely available, from high-throughput phenotyping technologies, repeated measures, or multi-environment trials. However, the vast majority of statistical models used in plant and animal breeding today handle only a single trait (or at most a few traits) at a time. We have developed a new statistical model for genomic prediction that efficiently and robustly scales to thousands of traits, allowing simultaneous predictions of high-dimensional phenotypes using data from complex experimental designs. Our approach overcomes the computational bottlenecks and over-parameterization challenges of traditional multi-trait linear mixed models by combining recent innovations in computational algorithms and statistical theory. These include: i) efficient and tunable Bayesian priors that prioritize only the strongest, most informative signals in Big Data, ii) a latent factor structure for trait covariances, and iii) efficient approximation and implementation schemes for reducing computational costs in mixed models. We will demonstrate the utility of our approach in the context of incorporating hyperspectral imaging data in the evaluation of wheat lines for grain yield.